Version 1.3 is now available!
RADERON AI LAB
DATASTUDIO
Artificial Intelligence Laboratory
Login To DATASTUDIOGet Demo
By using Raderon Lab DATASTUDIO, you agree to its license and privacy statement.
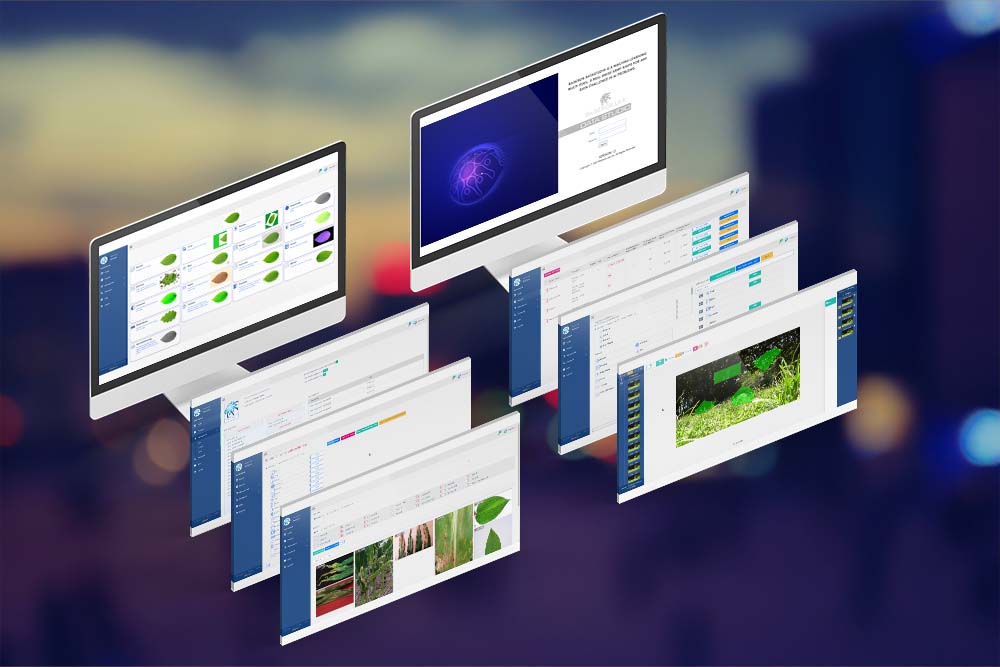
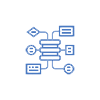
Image Gathering
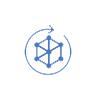
Image Augmentation
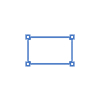
Image Annotation
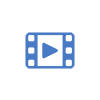
Video Annotation
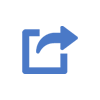
Export
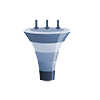
Pipeline
![]() Image Gathering |
![]() Image Augmentation |
![]() Image Annotation |
![]() Video Annotation |
![]() Export |
![]() Pipeline |
Raderon AI Lab DATASTUDIO
Getting your hands on datasets quickly to test algorithms and develop models has become a critical skill for today's data scientists and machine learning engineers. It is equally vital to go from a semi-complete dataset to one that is fully annotated, fleshed out with additional enhanced data, and ready to move on to model creation.
We designed the DATASTUDIO tool to assist with data gathering, annotation, and augmentation chores for those who have incomplete data and need some help taking it from "not quite" to "let's go!"
Using DATASTUDIO will access the highest-quality annotation of picture and video data for complex models. Ideal for computer vision models, sentiment analysis, entity linking, and syntactic parsing and tagging.
Our tools enable you to annotate your photos and videos faster, smarter, and better by providing a comprehensive collection of capabilities to equip all of your computer vision labeling tasks. The Raderon Lab DATASTUDIO Tool is intended to increase productivity and scale.
Connect to many sources, apply filters, and build project-specific data subsets. Set up labels for your dataset. Choose from multi-selection types such as rectangle and polygon, and finally export labeled data to the format you require, such as CSV, COCO, Pascal VOC,YOLO , etc.
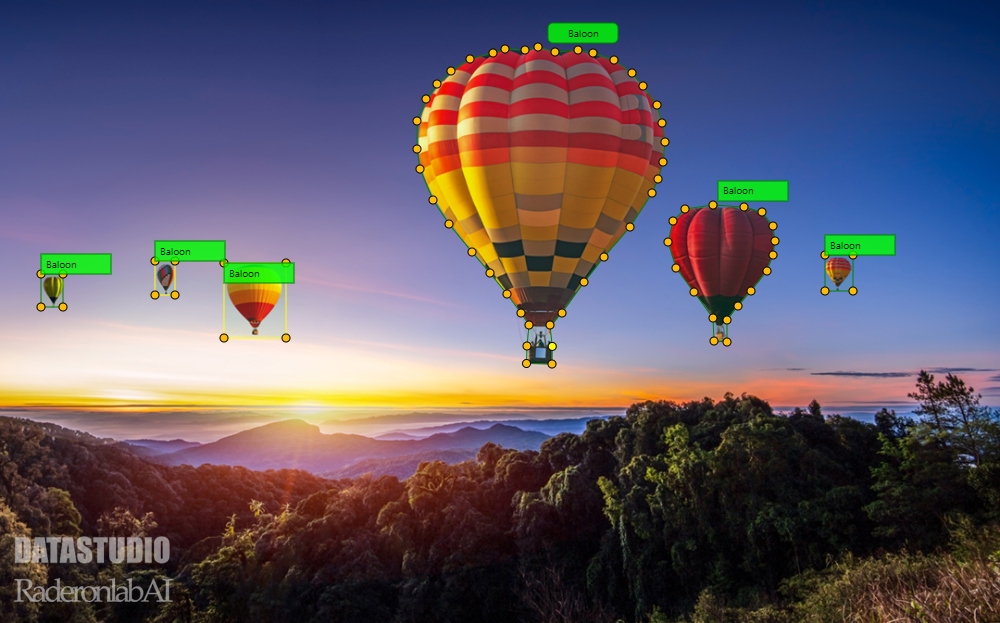
Image Gathering
Gather raw data for augmentation and annotation activities.
One of the essential parts of machine learning applications is data collection.
Because machine learning models are now widely used, having massive datasets on a domain-specific job does not guarantee higher performance.
The model's performance is determined by the dataset's quality and labeling/annotation.
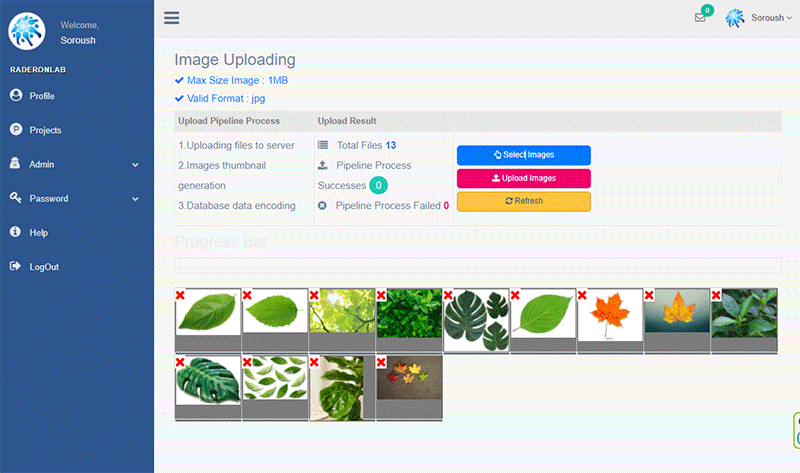
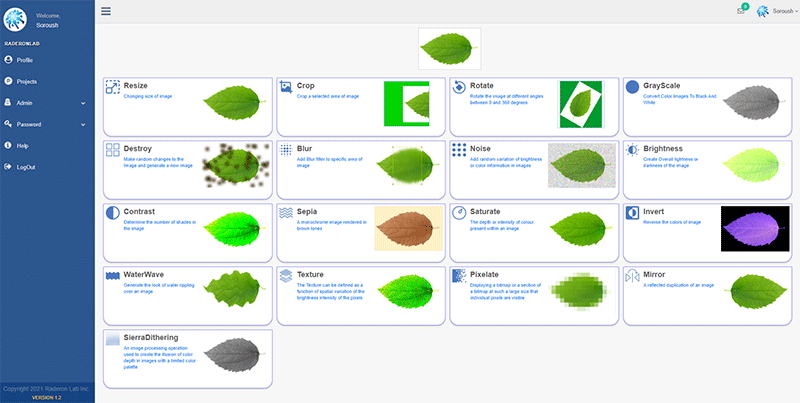
Image Augmentation
Image augmentation is changing or "augmenting" a dataset with new information.
This additional input might range from photographs to text, and its inclusion in machine learning algorithms improves their performance.
For example, suppose we wanted to develop a model to categorize dog breeds, and we have a large number of photos of all species except pugs.
As a result, the model would struggle to classify pugs. We could improve the data by including some (actual or false) pug photographs or increasing our existing pug images (e.g., by replicating and distorting them to make them artificially unique).
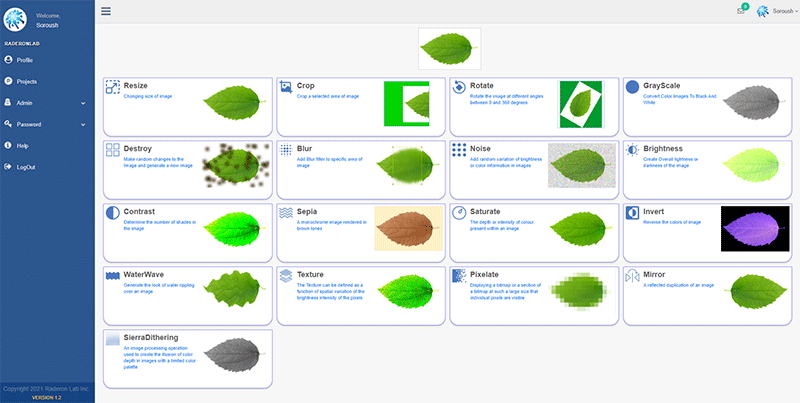
Image Annotation
To construct an AI or ML model that performs like a human, training data is required.
A model must be trained to grasp specific information to make judgments and take action. Annotating data for AI applications is the process of categorizing and labeling data.
Each use case of training data requires classification and annotation. Businesses can design and improve AI solutions with high-quality, human-powered data annotation.
To understand images, image annotation is critical for many applications, including computer vision, robotic vision, facial recognition, and machine learning systems. To provide this information, metadata, such as identifiers, captions, or keywords, must be added to each photograph.
Image Annotation Rectangle
Image Annotation Polygon
Many use cases necessitate large amounts of annotated photos, ranging from self-driving automobiles and machines that pick and sort produce to healthcare applications that auto-identify disease disorders. By efficiently training these systems, image annotation improves precision and accuracy.
Image Annotation FREEHAND Polygon
Video Annotation
Video annotation is the technique of labeling or tagging video clips to train computer vision models to detect or identify things.
Unlike image annotation, video annotation entails tagging objects frame by frame to make them recognizable to machine learning models.
Ground truth datasets are generated through high-quality video annotation for optimal machine learning capabilities. Deep learning applications for video annotation can be found in various areas, including self-driving cars, medical AI, and geospatial technology.
Formats for Annotation Export
The most prevalent label formats for computer vision are supported by DATASTUDIO. Choose between COCO, Pascal VOC, YOLO, Create ML, or our own JSON format to export.
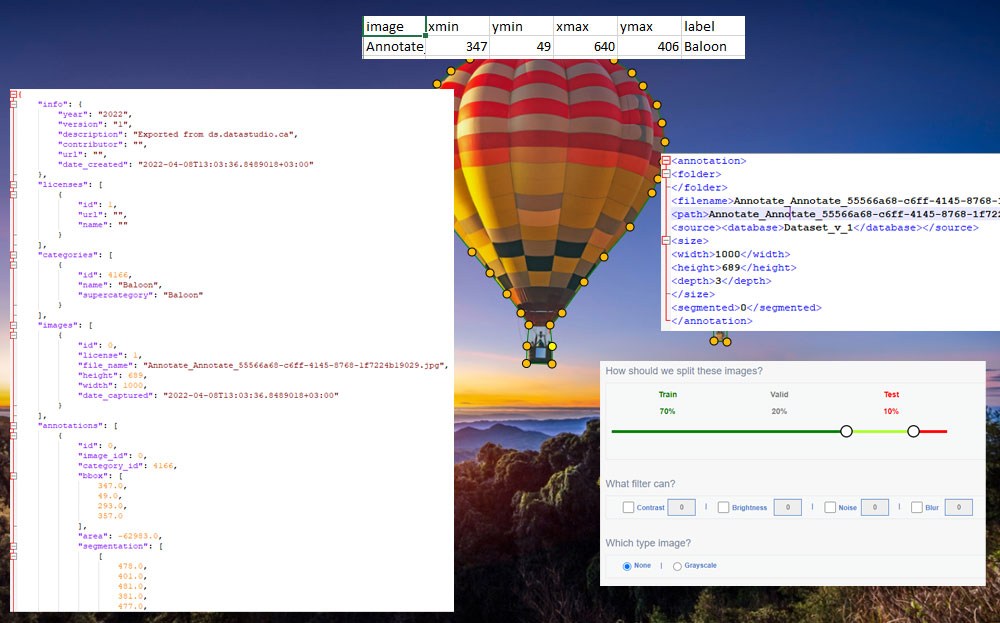
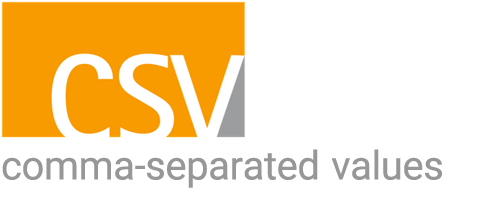
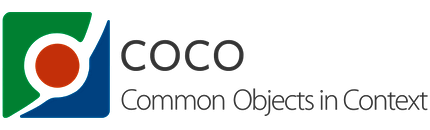
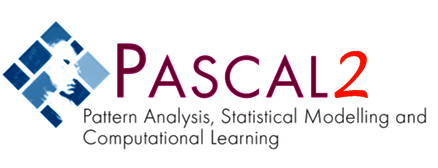
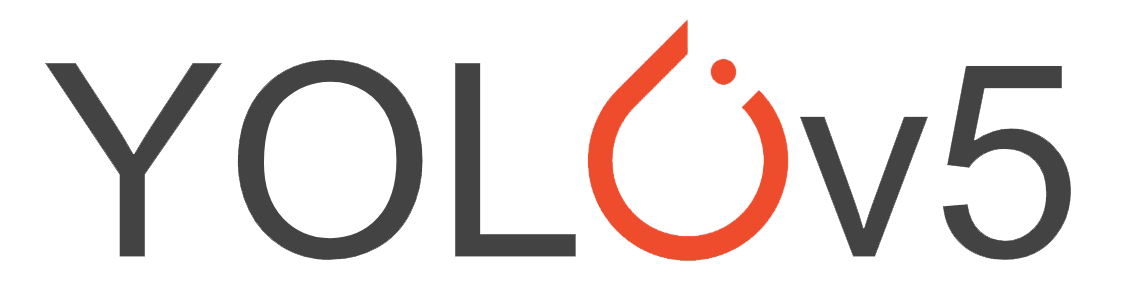
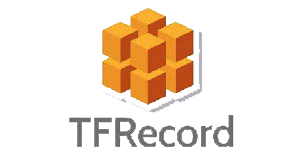
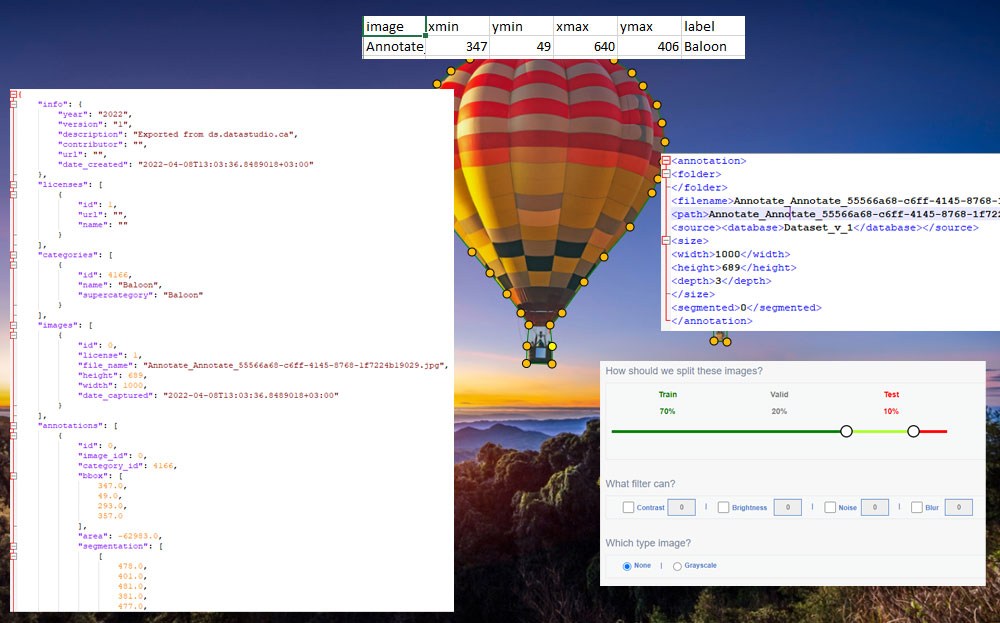
Pipeline
You can use the pipeline tool to establish sequential data augmentation steps that make copies of existing images with some alteration.
We can, for example, cut and rotate an image to make it appear new to our training model. Using this ensembling strategy, we may multiply our dataset and train the model to increase its accuracy.
The pipeline tool is convenient and straightforward, with a wide range of DATASTUDIO augmentation tools available. You can create different pipeline packages, save and load them for any dataset, and modify them for individual datasets.